The matrixes below detail the areas in AI where banks and insurers should focus their time and resources. We suggest that companies invest in technologies shaded in green, explore the prospect of investing in technologies shaded in yellow, and ignore areas shaded in red.
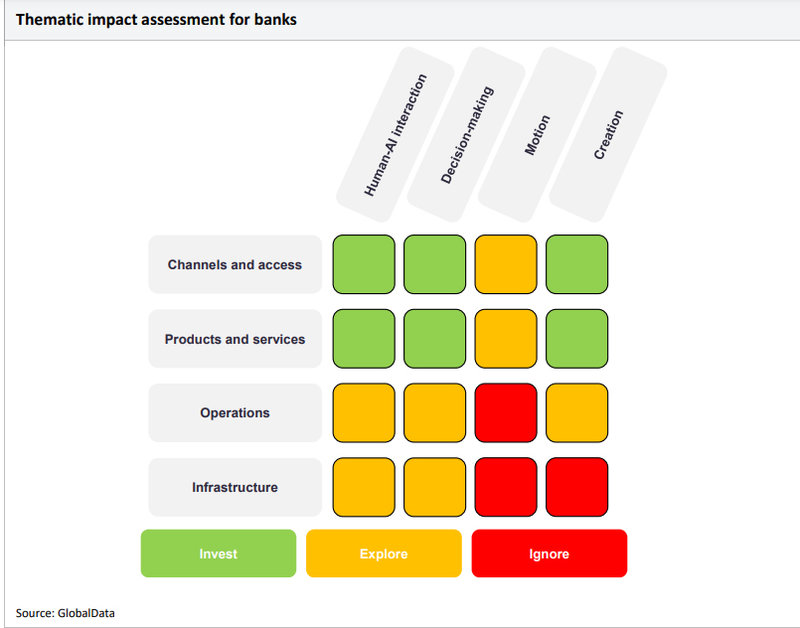
We recommend banks invest in human-AI interaction, decision-making, and creation across the channels and access and products and services layers of the banking value chain. The most common and advanced human-AI interaction examples are CV and conversational platforms. Conversational platforms are a necessary area of investment for banks in the channels and access segment of the value chain as, with the shift to digital banking, more customers demand round-the clock support.
In the long run, banks will be better served by the comprehensive deployment of virtual assistants rather than relying on staff. Banks can either invest in proprietary technology or, more feasibly, partner with a tech vendor and then integrate and tailor the product to their systems. CV is another area that is predominantly relevant on the customer side, as it is mainly used as a biometric method to secure accounts.
Banks should also focus on the decision-making segment, as AI can be used to improve market trends forecasts and price predictions. The creation category, also known as generative AI, includes anything related to AI creating content in any shape or format. Financial institutions are increasingly exploring use cases of generative AI, and it will be beneficial for them to invest in the technology across the channels and access and products and services layers of the banking value chain.
Generative AI can play a critical role in portfolio management by providing market analysis, risk assessment, portfolio optimisation, scenario analysis, investor communication, incorporating behavioural finance insights, and applying ML techniques. It can assist in analysing market trends, evaluating risks, optimising portfolio allocation, simulating scenarios, communicating with investors, addressing behavioural biases, and utilising historical data for predictive modelling.
How AI helps resolve the challenge of personalisation
Financial institutions can use AI to create more personalised products and deliver tailored services to their customers. AI algorithms can analyze vast amounts of customer data, including behavior patterns and transaction history, to understand customer preferences and make customised product recommendations.
Behavioural analytics are also extremely useful in gaining insights into customers’ financial goals and spending habits to personalise marketing campaigns and promotions to match customers' interests and needs. Some financial institutions are already enhancing their customers' digital experiences through advanced AI-driven solutions for personalisation.
For example, in March 2023, Visa collaborated with AI and big data company Crayon Data, enabling card issuers to use AI-driven insights to enhance customer engagement through hyper-personalisation. Crayon Data's AI platform gathers data across diverse lifestyle categories to construct a graph-based entity-affinity model called Tastegraph.
This model is subsequently linked with Visa’s customer behaviour data, enabling the exploration of a wide range of choices and personalised recommendations. Issuers aim to generate additional revenue by offering unique solutions to cardholders and merchants.
Financial institutions can also use AI-powered chatbots and virtual assistants to offer personalised customer support. Using ML, chatbots can continuously improve their responses to customer inquiries and tailor interactions based on individual customer preferences. Banks and insurers alike have been adopting virtual assistants to provide personalised guidance to their customers, improving their customer relationships while streamlining their operations.
Big data visualisation can help financial institutions apply AI for better risk assessments and credit scorings. In the insurance sector, AI-powered data analytics and risk assessment tools will allow insurers to create real-time and adaptive profiles of their customers, which helps design products that more closely fit customers’ needs and behaviours. Breaking down products into further granularity to provide customisable coverage rather than a one-size-fits-all approach will help insurers retain customers.
How AI helps tackle the challenge of cybersecurity and fraud risks
Financial institutions hold some of the most important and sensitive personal data: not just names and addresses but also direct access to financial data, savings, and investments. Therefore, they must protect this data from both malicious entities and outright losses. AI is an important tool in the battle against ever-evolving cyber threats.
For example, ML can identify the normal pattern of behaviour for customers and raise the alarm if there is a deviation from this norm. This may prompt manual intervention or the threats may be automatically countered. A financial institution should identify and combat breaches early, ensuring robust security and mitigating reputational damage.
Identity management tools that incorporate computer vision technology can enhance robust preventative cybersecurity. This has become widespread with the use of facial recognition in smartphones, such as Apple’s Face ID. These tools add an extra layer of security to mobile apps, an increasingly popular channel for banking access. A facial recognition barrier makes it more difficult for criminals to hack into customer accounts.
Fraud and money laundering are other major challenges financial institutions face; failure to identify can result in hefty fines. To meet compliance requirements and avoid fines, financial institutions can adopt AI-driven anti-money laundering (AML) solutions.
In 2018, HSBC partnered with AI tech start-up Ayasdi to develop an AI-enabled AML solution that analysed customer payment sources and destinations to ensure funds were coming from legitimate sources and detect deviations from preestablished norms.
Ayasdi’s AI solution allowed HSBC to identify fraud and stop payments before they violated regulations. The solution helped HSBC to automate AML investigations and reduce its false positives by 20%.
How AI helps resolve the challenge of channel shift
Customers increasingly prefer digital channels for their financial needs. AI can help financial institutions adapt and provide seamless experiences across various channels, including mobile apps, websites, chatbots, and social media platforms. Financial institutions must improve customer engagement and loyalty to avoid losing customers during this channel shift.
Financial companies can better navigate channel shifts by offering personalised experiences, streamlining processes, and using data-driven insights to optimise digital channels. For instance, chatbots can provide 24/7 support to customers through digital channels, meeting customer expectations for immediate and convenient service. Natural language processing (NLP) incorporated into chatbots allow automated channels to deal with most customer queries without the need to escalate numerous cases to humans.
Fintechs, in particular, have benefited from the shift from in-person to online channels. In March 2023, American fintech Galileo launched Cyberbank Konecta, a conversational AI platform for fintech, financial institutions, and non-financial brands. Cyberbank Konecta’s AI platform can help it improve customer engagement and loyalty with 24/7 customer support in any language across every channel, and the AI engine gets better with each customer interaction. The platform can also help reduce operational and service costs by managing 80% or more customer inquiries with digital assistants. By automating manual and repetitive tasks, AI can be used effectively to improve the speed and efficiency of digital channels.
How AI helps tackle the challenge of ESG
Financial institutions can use AI algorithms to analyse vast amounts of data related to environmental, social, and governance factors and assess the risks associated with lending, investment, and underwriting activities.
ESG-related data, like carbon emissions, diversity and inclusion metrics, or corporate governance practices, can efficiently be analysed during this process, and financial institutions can use this analysis to score companies based on their ESG performance. These can then be used to create risk profiles and identify and mitigate potential ESG risks more effectively.
AI can also help financial institutions streamline the process of ESG reporting and compliance by automating data collection, analysis, and reporting. Using NLP and ML, AI algorithms can obtain relevant ESG information from corporate reports. Financial institutions can then generate comprehensive ESG reports, which comply with regulatory requirements and provide transparency to stakeholders.
As people become more mindful of the issues society faces, like climate change, impact investing has become increasingly popular worldwide. This alludes to investments made into companies that aim to add to social good – whether that’s decreasing carbon emissions or building houses for those in need. AI can help investors manage their portfolios' social impact by combining big data and proprietary impact methodologies for sustainability analysis.
In addition, where comprehensive research and data on sustainable investment options may not be widely available, making it challenging for investors to undertake extensive due diligence before investing, AI can enable quick access to data and ESG performance screening capabilities
By applying ML algorithms to appropriate ESG criteria, AI can evaluate many companies quickly and accurately. An example of a bank using AI in such a way is JPMorgan, which launched Sustainable Investment Data Solutions for institutional investors in June 2023.
How AI helps tackle the challenge of the cost-of-living crisis
AI can be employed to tackle the challenges faced by financial institutions due to the cost-of-living crisis. Chatbots and virtual assistants can provide customers with immediate support and reduce admin hours, cutting company costs. Banks can use AI-powered financial management tools to help their customers navigate their finances and expenses.
For example, fintech Plum uses AI technology to automatically evaluate users’ expenditure and calculate affordable amounts to save. It analyses customer transaction histories and uses an algorithm to adjust automated savings to current spending levels. Users can withdraw savings and also set limits on the amount saved. RBC also uses NOMI Budgets, an AI and predictive analytics solution integrated with the RBC mobile app, to track the spending patterns of customers. It then provides personalised financial insights based on various spending categories, such as shopping, cash withdrawals, entertainment, transport, and dining.
Insurers can use AI algorithms incorporating data such as credit scores, lifestyle habits, and geography to assess the risk profiles of policyholders and personalise offers, pricing, and recommendations. This, in turn, can lead to greater customer satisfaction and cheaper premiums. Better risk mitigation strategies can also be developed using AI, encouraging customers to adopt behaviours that minimise risk and reduce the chances of expensive claims.
GlobalData, the leading provider of industry intelligence, provided the underlying data, research, and analysis used to produce this article.
GlobalData’s Thematic Intelligence uses proprietary data, research, and analysis to provide a forward-looking perspective on the key themes that will shape the future of the world’s largest industries and the organisations within them.